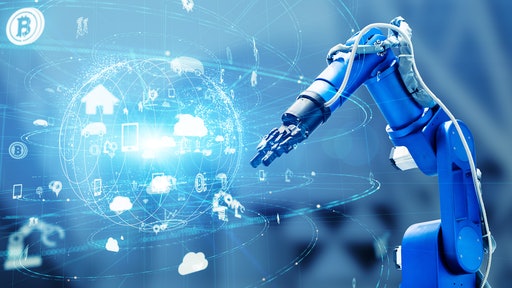
Machine learning originated in the 1980s. Although it is still old in modern terms, it is significantly more progressive than AI. Machine learning is steeped in Artificial Intelligence Trends techniques that allow computers to learn patterns and behaviors without explicit programming. This is a big problem. Just as the value of a new car is constantly changing as soon as it is taken off the lot, so is the data.
Due to this perpetual change, predefined rules such as if / then statements can become less effective or increasingly obsolete as time passes if they are not adhered to. In other words, Machine Learning Application algorithms are continually working to achieve an optimal probability that predictions are correct as the data evolves. As newer and more recent data is added to the model, the optimization is dynamic.
Why AI / ML?
Intent-based networks provide easier operations for today’s complex software-defined networks. But, as these networks grow more and more, the great programming capacity of the devices and the flexibility in their configuration leads to unimaginable levels of complexity. A network analytics engine, powered by artificial intelligence and machine learning (AI / ML), is simply the only way humans can navigate this complexity. This short manual will explain why we need AI / ML, the basics of AI / ML related to network analysis, and the role they play in intent-based networks.
First, let’s take a look at the challenges IT teams face today:
There is a proliferation of client devices that connect to the network, such as laptops, smartphones, cameras, sensors, machines, robots, thermostats, lighting, etc. Each of these client devices requires a different set of parameters to be configured on the network in order to ensure user experience and security. The Cisco Visual Networking Index predicts by 2019 that there will be, globally, 28.5 billion devices and network connections by 2022 (that is, three times the human population).
Users are moving towards wireless technology as the primary means of network connectivity on campus. Wireless communications are much more complex in terms of the number of parameters that must be configured to ensure an optimal user experience. Additionally, the wireless environment is highly dynamic, and performance can vary depending on the number of users, services, and applications, and levels of interference.
Applications are moving to the cloud. The network architecture needs constant updating to support the different data entry points on campus and the various paths that the data will travel.
The goal of Future Artificial Intelligence and Machine Learning intent-based networking is to automate the network to dynamically meet business intent and priorities; the network is constantly learning and adapting to safely maintain business intent. First, business intent is translated into a policy based on the identity of the user or device and the application requirements for that job or device role.
For example, a salesperson’s job requires quality, reliable video conferencing, finance may require low-latency connectivity to an overseas banking database, and a factory emergency shut-off valve would require the instantaneous transmission of very low amounts of data. These policies are then translated into network infrastructure configurations and implemented through controller-based automation throughout the network. Finally, the network constantly monitors, collects data, and analyzes to make sure the business intent is being met.
Supporting these new challenges means collecting information in the form of real-time telemetry across the entire network, in order to track key performance indicators (KPIs) and identify anomalies — that is, things with poor performance on the network. But the amount of anomalies and alerts that are generated is simply too much to be useful. Today’s networks are generating massive amounts of data, and this means too much noise for humans to handle in a timely manner. AI / ML can learn to differentiate between important alerts and trivial anomalies, thereby reducing this noise.
Why Machine Learning Will Change Business Software
Every day the business faces challenges from administration to operations. Business software has so far played an important role in addressing many of these vital processes. But the growing demands on business meant that a newer approach is needed to deal with the various fields. With the introduction of machine learning in the enterprise, a window of possibilities was left open.
Machine learning provides the right framework that companies can trust. The algorithms and Applications of Artificial Intelligence and ML that are used in an embedded machine learning computer system can provide predictive insights by leveraging the huge data sets that companies own. This data includes structured and unstructured forms, such as databases and the Internet.
The shift to Machine Learning Software Projects has clearly benefited organizations by providing improved and faster data insights and analytics. It can improve the efficiency of internal operations within an organization along with a better understanding of the customer.
When it comes to internal operations, a clear use case for business software with machine learning is the area of logistics. An ML system can automate the processes involved in managing orders, inventory, shipping, and warehousing by making data-driven decisions without human intervention.
The Importance of Framing, Scoping, and Defining Problems in Machine Learning Models:
Phew! We’ve gotten over the technical side of things and are now into one of our favorite aspects of machine learning and predictive modeling: framing the problem you intend to solve.
Organizations aspire to build and implement a multitude of machine learning models. Because there are so many, it can be difficult to know where to start. Some people argue that it is best to start with the top business initiatives or most critical metrics. While it is valuable to have identified a model, we argue that you need to dig deeper by asking more questions early on, such as:
- How do you think to identify the goal metric for which you want to predict?
- How do you identify the disadvantage of the target metric?
- What level of context and breadth of data can it include?
- Is there any third-party data that we can leverage to add incremental value to the model?
Also Read: Difference B/W Artificial Intelligence and Machine Learning
How can we assimilate the knowledge (both from development and deployment) of our model into the appropriate organizational communication pathways, processes, and materials/literature to enable digital economies of scale?
It is imperative that you frame a question or objective in a way that is conducive to predictive modeling. After determining that you have a problem with high customer churn, what are your next steps? Yes, you want to create a model that helps you understand which customers have left and predict which customers are most likely to leave, but before you can do so, you must define churn.
As with many elements of the modern marketing funnel, turnover will vary by the business model. By definition, churn can occur in a variety of transactions, such as regular product purchases, hotel visits, vehicle maintenance, or subscription renewal. No matter the case, the specific definitions and parameters of that given model are imperative. With proper data preservation and configuration, the ability to create and implement accurate and relevant models increases. Additionally, ensuring that the right stakeholders across the business are involved in this part of the modeling process will make the results more relevant and increase the chances of adoption as the process matures.
Machine learning experiment:
Data Scientists — In many organizations, machine learning engineers and data scientists tend to work alone. That makes some people think that keeping track of their experimentation process isn’t that important as long as they can deliver that latest model. This is true to an extent, but when you want to go back to an idea, rerun a model from a couple of months ago, or simply compare and visualize the differences between runs, the need for a system or tool to track ML experiments becomes (painfully) apparent.
Teams of Data Scientists — A specialized tool for tracking machine learning experiments is even more useful for the entire team of data scientists. It allows them to see what others are doing, shares ideas and knowledge, stores experiment metadata, retrieves it at any time and analyzes it when needed. It makes teamwork much more efficient, avoids situations where several people work on the same task, and makes it easier to join new members.
Managers / Business People — Tracking software creates an opportunity to engage other team members like managers or business stakeholders in your machine learning projects. With the ability to prepare visualizations, add comments, and share work, managers and coworkers can easily track progress and cooperate with the machine learning team.
Cost savings of using machine learning:
Let’s calculate the salary costs of ML practitioners.
When hiring machine learning professionals, companies pay between:
- $ 40 and $ 75 per Machine Learning Solution Services developer per hour.
- Artificial Intelligence Development Company costs $ 40 and $ 80 per hour.
- $ 45 and $ 80 per data scientist per hour.
- $ 80 and $ 180 for DevOps per hour.
- Suppose the average AA practitioner works 40 hours per week and 52 weeks per year.
By reducing maintenance costs, organizations free up to 42.1% of the developer’s workweek.
With serverless machine learning, companies save between:
- $ 35,000 and $ 66,000 per machine learning developer per year.
- $ 35,000 and $ 70,000 per Cost of Artificial Intelligence developer per year.
- $ 39,000 and $ 70,000 per data scientist per year.
- $ 70,000 and $ 158,000 for DevOps per year.
USM Business Systems is one of the top companies for Artificial Intelligence Development, Human Resource Management Systems, Mobile Application Development, Chatbot Development, data quality solutions, workforce service to create interactive experiences for major platforms. USM also provides Artificial Intelligence in Retail and Artificial Intelligence in Manufacturing.
WRITTEN BY
Koteshwar Reddy
I’m a tech assistant. and content researcher at USM. I share my knowledge about information in modern technologies.