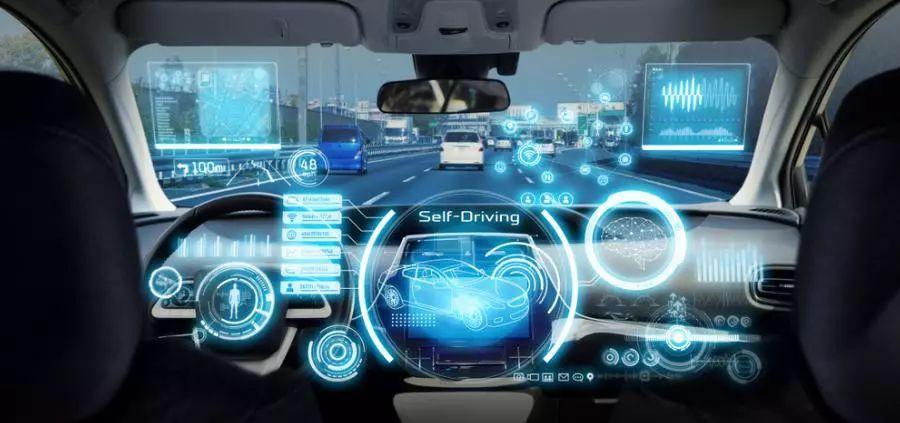
This article provides a brief and comprehensive overview of the key components of autonomous vehicles (autonomous driving systems), including autonomous driving levels, autonomous vehicle sensors, autonomous vehicle software, open source data sets, industry leaders, autonomous vehicle applications, and ongoing Challenges facing.
In the past ten years, many research papers have been published in the field of autonomous driving. However, most of them only focus on specific technical fields, such as visual environment perception, vehicle control, etc. In addition, due to the rapid development of autonomous vehicle technology, such articles quickly become obsolete.
In the past decade, with a series of breakthroughs in autonomous driving system technology worldwide, the competition for the commercialization of autonomous vehicles (automatic driving systems) has become more intense than ever. For example, in 2016, Waymo launched its own self-driving taxi service in Arizona, which attracted widespread attention. In addition, Waymo spent about 9 years developing and improving its autonomous driving system, using various advanced engineering technologies such as machine learning and computer vision. These cutting-edge technologies have greatly helped their driverless cars understand the world better and take the right actions at the right time.
Due to the development of autonomous driving technology, many scientific papers have been published in the past ten years, and their citations have increased exponentially, as shown in Figure 1. We can clearly see that since 2010, the number of publications and citations each year has gradually increased, and reached its peak last year.
Autopilot system
The autonomous driving system enables the car to operate in a real environment without human driver intervention. Each autonomous driving system consists of two main components: hardware (car sensors and hardware controllers, ie, throttle, brakes, wheels, etc.) and software (function group).
In terms of software, it has been modeled in a number of different software architectures, such as Stanley (Grand Challenge), Junior (Urban Challenge), Boss (Urban Challenge) and Tongji Autopilot System. The Stanley software architecture includes four modules: sensor interface, perception, planning and control, and user interface. The Junior software architecture consists of five parts: sensor interface, perception, navigation (planning and control), wire control drive interface (user interface and vehicle interface), and global service. Boss uses a three-tier architecture: task, behavior, and motion planning. The Tongji Autopilot System divides the software architecture into: perception, decision-making and planning, control and chassis. This paper divides the software architecture into five modules: perception, positioning and mapping, prediction, planning, and control, as shown in Figure 2, which is very similar to the software architecture of Tongji's automatic driving system.
Classification of Autonomous Driving
According to the Society of Automotive Engineers (SAE International), autonomous driving can be divided into six levels, as shown in Table 1. The human driver is responsible for the driving environment monitoring (DEM) of the 0-2 level automatic driving system. Starting from level 4, human drivers are no longer responsible for dynamic driving task backhaul (DDTF). At present, the most advanced autonomous driving systems are mainly in level 2 and 3. The industry generally believes that it may take a long time to reach a higher level of autonomous driving.
Sensors installed on autonomous driving systems are usually used to perceive the environment. Each sensor is selected to weigh the sampling rate, field of view (FoV), accuracy, range, cost, and overall system complexity. The most commonly used sensors are passive sensors (such as cameras), active sensors (such as lidar, radar, and ultrasonic transceivers) and other sensor types, such as global positioning system (GPS), inertial measurement unit (IMU).
The camera captures two-dimensional images by collecting light reflected on three-dimensional environmental objects. Image quality usually depends on environmental conditions, that is, different weather conditions and different lighting environments will have different effects on image quality. Computer vision and machine learning algorithms are commonly used to extract useful information from captured images/videos.
Lidar uses pulsed laser light to illuminate the target, and measures the distance to the target by analyzing the reflected pulse. Because lidar has high three-dimensional geometric accuracy, it is usually used to make high-resolution world maps. Lidar is usually installed in different parts of the vehicle to achieve different functions, such as the top, side and front. A more detailed introduction about Lidar
Radar can accurately measure the distance and radial velocity of the target by emitting electromagnetic waves and analyzing the reflected waves. Radar is particularly good at detecting metal objects. Of course, radar can also detect non-metal objects, such as pedestrians and trees in short distances. Radar has been used in the automotive industry for many years, giving birth to ADAS functions, such as automatic emergency braking and adaptive cruise control.
Similar to radar, ultrasonic sensors calculate the distance to the target by measuring the time between transmitting an ultrasonic signal and receiving an echo. Ultrasonic sensors are usually used for positioning and navigation of autonomous vehicles.
GPS is a satellite-based radio navigation system of the US government that can provide time and geographic location information for automated driving systems. However, GPS signals are easily blocked by obstacles such as buildings and mountains, such as the so-called urban canyons, where GPS often does not perform well. Therefore, inertial measurement units (IMUs) are usually integrated into GPS devices to ensure the positioning of autonomous vehicles in "urban canyons" and other places.
Hardware controller
The hardware controller of an autonomous vehicle includes a torque steering motor, an electronic brake booster, an electronic throttle, a gear lever, and a parking brake. The state of the vehicle, such as wheel speed and steering angle, can be automatically sensed and sent to the computer system through the Controller Area Network (CAN) bus. This allows human drivers or automated driving systems to control the accelerator, brakes and steering wheel.
Existing challenges
Although autonomous driving technology has developed rapidly in the past decade, there are still many challenges. For example, the perception module does not perform well in bad weather and/or light conditions or in a complex urban environment. In addition, most perception methods are usually computationally intensive and cannot run in real-time on embedded and resource-limited hardware. In addition, due to long-term instability, the application of SLAM methods in large-scale experiments is still limited. Another important issue is how to fuse the sensor data of autonomous vehicles to create more accurate three-dimensional semantic words in a fast and economical way. In addition, when people can truly accept autonomous driving and autonomous vehicles is still a topic worth discussing, which has also led to serious ethical issues.