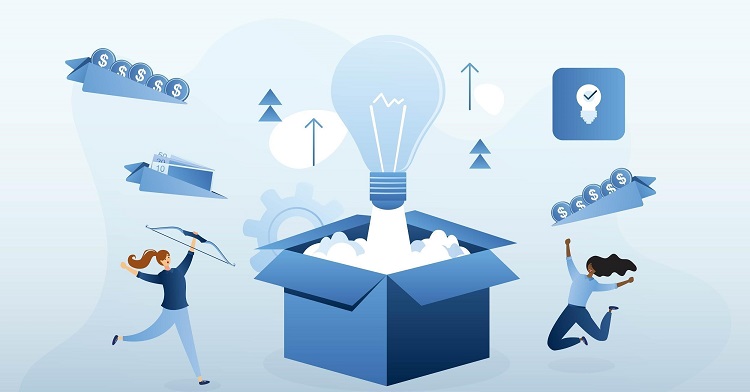
The new generation of AI chatbots requires complex neural connections to have conversations. This ability is scalable when developers use deep learning tools to help enterprises to bankroll AI-based intents with a high-tech approach. Unless the developer knows the pros, cons, and effect of deep learning tools on training chatbots, the very purpose of accurate deliverables gets lost in translation. click reference
Lessons to learn
Have you just met with failure in an AI Chatbot based project, recently? It is not success that teaches, but failure adds a valued experience while dealing with different approaches to creating chatbots. As many startups fail initially in their efforts, it becomes the ideal base for understanding how a developer can help an enterprise to monetize through deep learning tools.
Three things count:
- Deep learning does not involve or solve everything for business solutions. Some applications can do without it.
- All enterprises cannot deal with specialized tools unless they have the requirement.
- All developer tools are not meant for monetizing.
If an enterprise uses only deep learning tools, then only about 1/3rd of its potential will be realized and the rest will remain untapped. The developer needs an overall understanding to tap it.
Two systems for learning
An IT team will need to research AI Chatbots and its requirements so avoid aberrations related to conversations with humans and machines. Currently, virtual assistants like Cortana, Siri, and Alexa have set the bar for new bots. They work with smartphones, appliances, and other home-based devices. They work on 2 systems – Supervised learning and unsupervised learning which require natural language processing capabilities. According to Gartner by 2020, 85% of customers will be dealing with chatbots rather than human connections. And around the same time, medium and small enterprises will use product chatbots with satisfaction.
Supervised Learning
The software is developed after getting data from real-world requests. Correlations are established between ‘tags’ and ‘user-intents’ which are marked for learning and engaging the customer. In such a case, deep learning tools achieve a high level of accuracy. Specialized tools are developed for this purpose. The only hitch here is if the data collected is insufficient or not suitable then the functionality and success is trapped. go to this site
Unsupervised learning
Again, in this case, too, a good database is required to understand the customer intent by the chatbot. When it is not supervised, it works independently. There is no need for human supervision while it functions nor does it require specific tags to prompt it to work. The failure rate increases if the database does not provide a wide range of variables. The quality is not good enough for it to be released in the public domain. Even if it does come out, it will have limited success. The data volumes required are large for deep learning tools to be effective. And, it goes without saying that poor data does not give the required results. look here
Chatbots will continue to grow
Despite the failure rate, AI chatbots will grow and many companies will try to experiment with its capabilities. Consumers are already hooked on them and enjoy the services of such virtual assistants. They find an opportunity to add value to their routine tasks. Every public company wants to reduce customer care efforts, and this is a solution that has promise in the real world. The only reason why it fails in the case of an enterprise is that the data required for tags and user intent in each company is diverse and also limited to a certain extent. Hence, deep learning tools need careful deployment by the developer. They require a well-structured database and good examples for training the system. Getting advanced systems to work requires a degree of inference, latency, interpretability, and reproducibility to understand the data and train the program.
Developer’s skills are tested
A complex toolset may not be the answer to training a program to converse. It took years and several failed tests for Siri or Alexa to reach the stage where they are now. E-commerce giants using machine learning tools have survived as they have a constant flow of data to test and train. In the final analysis, a complete overview of components is required before they can be channeled and ready for public use or limited enterprise utility.
Last thoughts: If developers choose hybrid systems, advanced NLP, AI algorithms, and not rely on the 2 main systems there are bright chances of creating the right chatbot. additional info