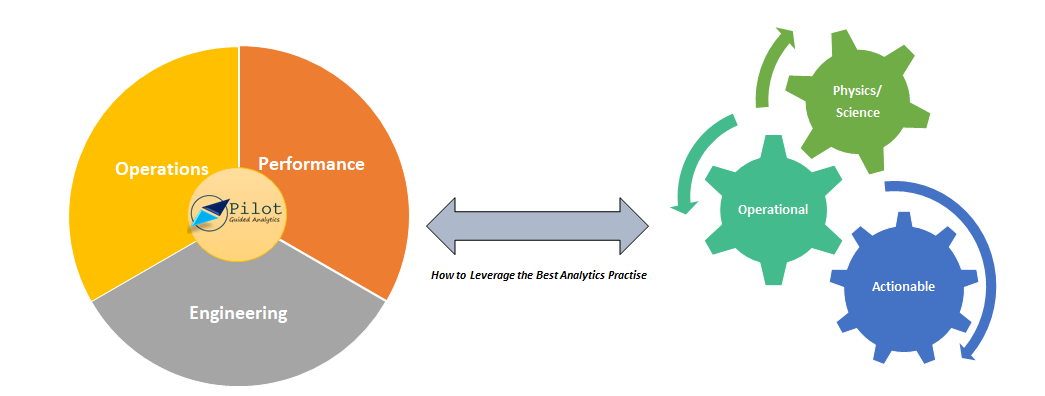
What is Soft-sensor?
Soft-sensor is a fancy term for the parameters which are derived as a function of the sensor- captured parameters. These could also be termed as “Latent Variables”, which are not readily available, but is required as a key metric to estimate the process/asset performance.
Read More @ https://dataanalytics.tridiagonal.com/soft-sensor-intelligence-to-operationalize-your-industry-oil-gas/
How to derive a Soft-sensor?
This question becomes important in-order to devise a right metric to derive the soft-sensor. These parameters could either be estimated through data-driven techniques or through first principle models.
Typically, we leap through the following steps to generate a soft-sensor:
- Select/Evaluate the right soft-sensor which is required to estimate the performance
- Gather all the required constants or the sensor signals which will be required to develop it.
- Look for the possible ways (Data-driven/Mechanistic) to evaluate it
- Test it in the production environment, and estimate it’s direct inference in the real-time operations
- If satisfactory, then use various forecasting rules/techniques to estimate the performance in the successive events
Soft-Sensor in Oil and Gas Industry
Soft-sensors has recently observed a lot of attraction in Oil and Gas industry. It’s been used heavily for assessing the performance of the industry enablers/assets such as Heat Exchangers, pumps, compressor.etc. Deriving such parameters becomes hard when we try to implement it in a process which is heavily governed by the first-principle/mechanistic models. This also brings in the requirement of integrating those models into the analytics workspace for a convenient and reliable analysis.
Let us look into the two different scenarios(Use cases) where we will be using soft-sensors derived through 1) Advanced Machine Learning Techniques, and 2)Mechanistic Techniques:
Use case I:
Debutanizer column performance prediction:
For debutanizer column performance is dictated by the extent of separation that happens to obtain a high purity C4 fraction from the tops as LPG (gasoline is obtained as the bottoms).
The challenge with this process comes when the gas chromatograph which is used for real time monitoring of the C4 fraction in the stream is off-due to maintenance. Such situation handicaps the operator/engineers to qualify the process performance. The lab analysis for estimating the quality of the product takes a while(few hours) to verify the process performance that happened in the past. This shows that gas chromatograph becomes a necessity to evaluate the process performance in real time, but due to its own maintenance challenges it brings in a major requirement to replace it with the possible mathematical model that can operate for all time without any operational failure in it’s own. This mathematical model can also be a potential replacement for lab analysis if developed with all the required parameters and assumptions.