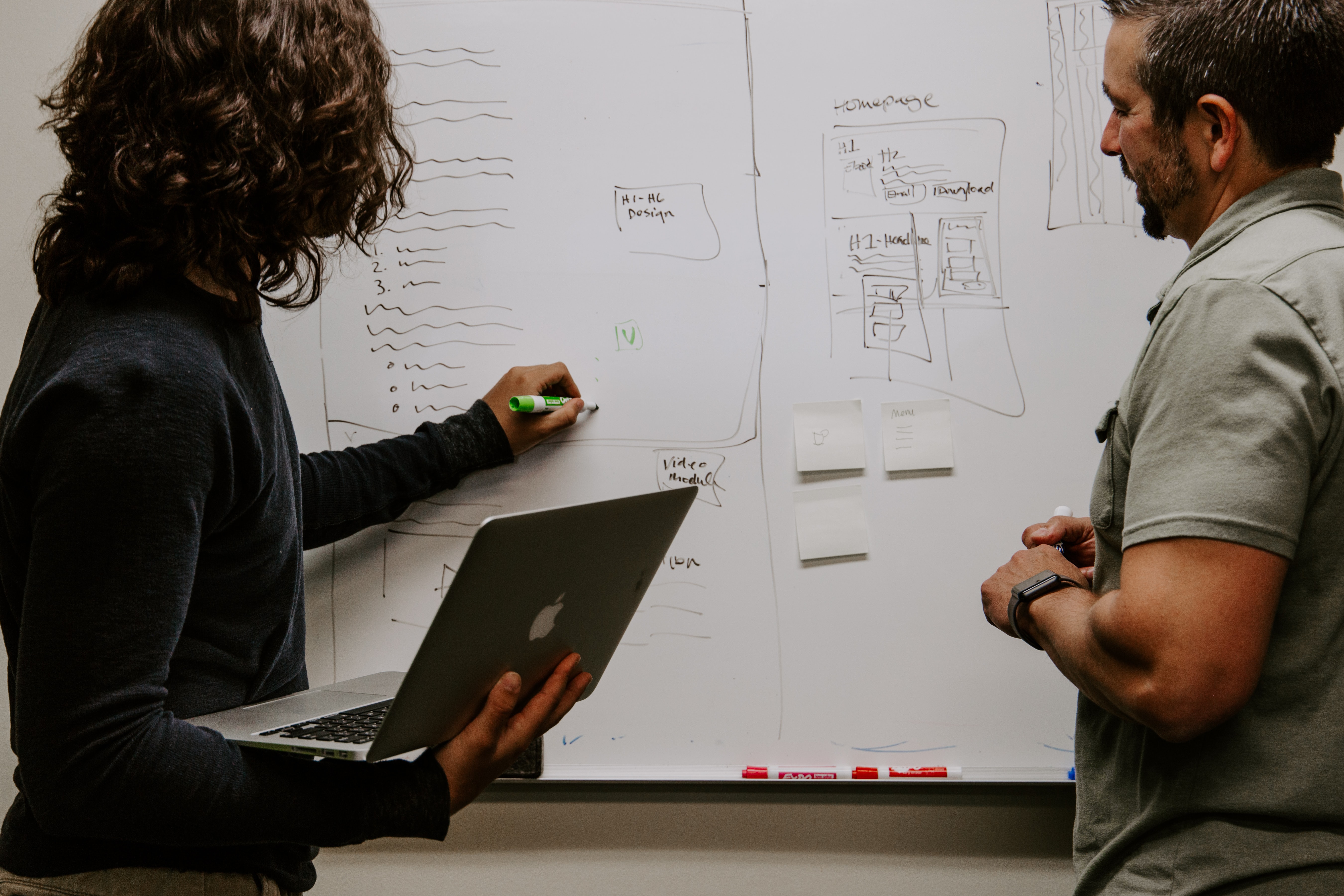
Each of the p unit vectors in the real coordinate space has a direction I that best fits the data and is orthogonal to the initial I 1 i-1 i-1 unit vectors for purposes of classification. The best-fitting line is the one with the smallest average squared distance between the points and the line. These axes come together to produce an orthonormal basis, where the linear dependence between data points is eliminated. In order to implement a change of basis on the data, PCA may be used to calculate the major components and then apply those findings to build statistics.
The best machine learning course online can enhance your skills.
When considering a set of p display-style p variables, the greatest amount of variance is explained by a linear combination of the original variables. To account for the remaining variation after the first component's effect has been subtracted, p-distribution-style iterations may be performed until the second principal component provides a satisfactory explanation. Since highly correlated variables are more difficult to work with, principal component analysis (PCA) is often used in these situations.
PCA is useful as a data exploration tool and a predictive modeling tool. It is common practice to "project" each data point onto just the top few major components of a dataset. This helps to decrease the number of dimensions in the dataset. The first PC, for instance, may be thought of as a route that maximizes the predicted degree of unpredictability. The variance of the projected data may be minimized by treating the principal components as orthogonal directions to each other, with the i-th component being the orthogonal direction to the first.
For either scenario, it is possible to show that the primary components are eigenvectors of the covariance matrix of the data. As a result, the primary components are often identified by means of either singular value decomposition of the data matrix or eigen decomposition of the data covariance matrix. One of the simplest eigenvector-based data analysis techniques is principal component analysis (PCA). Factor analytics, which employ a new matrix to solve the Eigenvectors of eigenvectors, is a technique that often makes more domain-specific assumptions about the underlying structure. Connected to principal component analysis (PCA) is canonical correlation analysis (CCA) (CCA). When analyzing data, principal component analysis (PCA) generates a new orthogonal set of coordinates to represent the variance within a single dataset, whereas canonical correlation analysis (CCA) generates a set of coordinates to explain the cross-covariance between two datasets. Strong, L1-norm based variants of the conventional PCA have also been proposed.
History
It was first proposed by Karl Pearson in 1901, but it wasn't until the 1930s that Harold Hotelling, working on his own, improved upon it and rechristened it the main axis theorem in mechanics. The Hotelling transform in multivariate quality control, the proper orthogonal decomposition (POD) in mechanical engineering, the singular value decomposition (SVD), the eigenvalue decomposition (EVD), and the factor analysis (for a discussion of the differences between PCA and a factor analysis) are all names for the same thing: the discrete Karhunen-Loève transform (KLT).
The machine learning course online can be helpful to get better insight on this topic.
Intuition
To get insights into how PCA fits an ellipsoid to data, imagine that each of the ellipse's axes stands for a different major component. Since the ellipsoid's axis is so small, there is very little motion in it.
The ellipsoid's axes may be found by subtracting the mean of the observed values from each of the observed values to center the values on zero. The adjusted values for each variable are substituted for the original ones. Next, we build covariance matrices for the data and determine their eigenvalues and eigenvectors. Then, the orthonormal eigenvectors need to be transformed into unit vectors. Following this, each of the symmetric unit eigenvectors may be interpreted as representing a unique elliptic curve that gives a perfect fit to the data. In this case, the variance of each axis will be represented by the diagonal elements of the covariance matrix. A rough approximation of the contribution of each eigenvector to the overall variance may be obtained by dividing the proportion of variation attributed to each by the sum of all values. Biplots and scree plots are used to talk about the PCA findings (the degree of explained variance).
The best online data science courses can give you a better understanding of this subject.