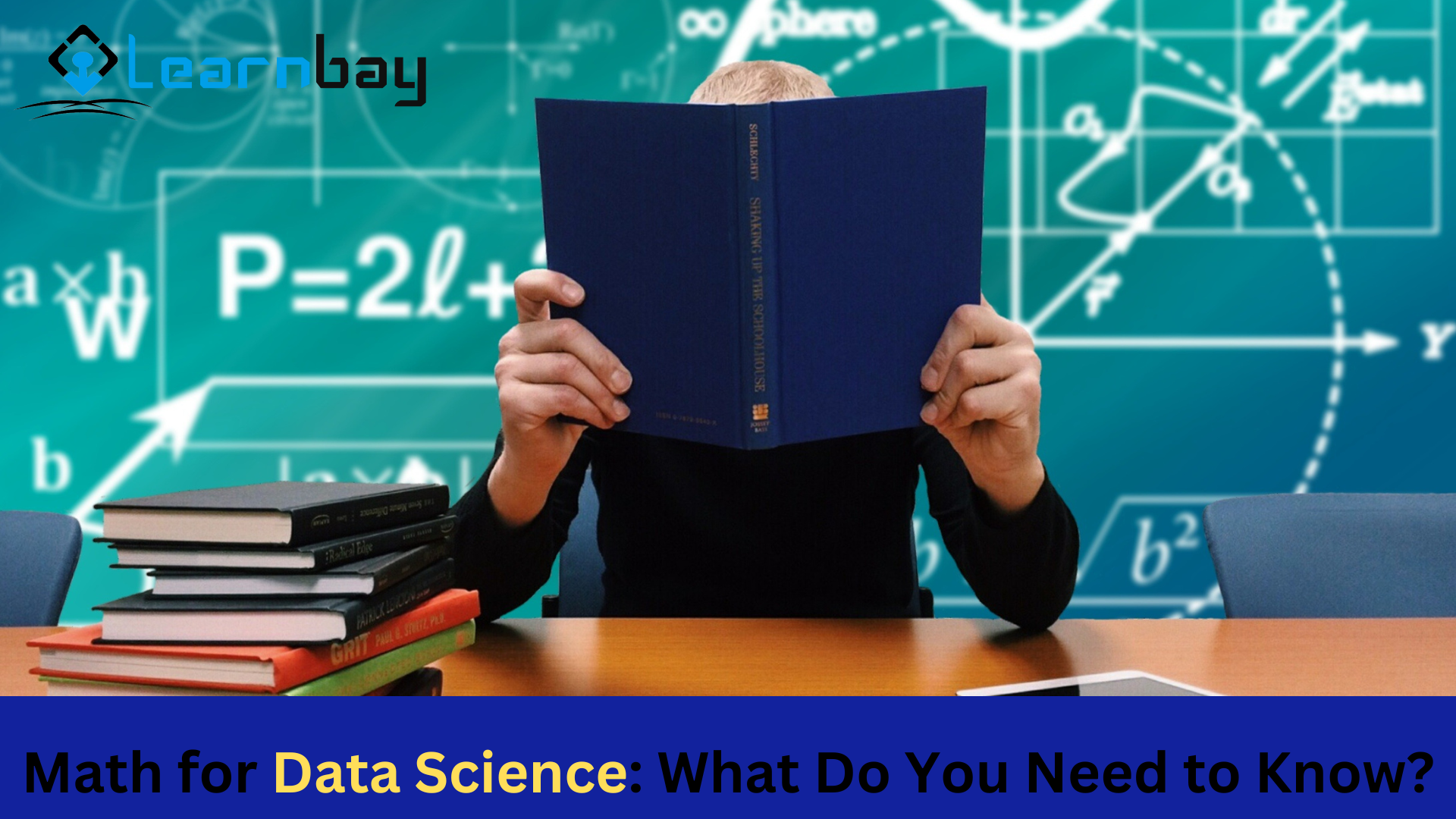
Data science depends heavily on mathematics. Thus, any person who wants to pursue a career in data science or is already a practicing data scientist must have a solid foundation in a few key areas of mathematics.
For most businesses, you will need at least a bachelor’s or master’s degree in math depending on your choice of job as a data scientist. Your success and knowledge of mathematics rely to a large extent on your capacity to apply your data science expertise to real-world situations.
Mathematical studies are required for careers in data science because machine learning algorithms, data analysis, and data deriving insights from data all entail arithmetic. Math is not the only need for a degree in data science, but it is sometimes the most important. It is generally agreed that one of the most crucial tasks in a data scientist's workflow is to recognize, understand, and translate business difficulties into quantitative ones. Join the best data science course in Chennai to gain a solid foundation on basic and advanced data science technologies.
Do you want to pursue a career as a data scientist, engineer in machine learning, business intelligence developer, data architect, or in another specialized field? Perhaps you aren't quite sure what direction your data science career will go. Consider the many kinds of mathematical criteria, though, and how data science implements them. You will be able to follow your chosen path in mathematical education better since you will better grasp your abilities and interests.
In order to provide you with a better understanding of the sorts of mathematics utilized in data science, let's start by looking at them. This will help you determine what you need to know about math for your data science career.
Math For Data science – What all you need to know
Here are a few of the most typical sorts of arithmetic you'll encounter in data science.
Linear algebra
Creating machine learning algorithms requires a solid understanding of how to construct linear equations. They will be used to analyze and track data collection. In loss functions, regularization, covariance matrices, and support vector machine classification in machine learning, linear algebra is applied.
Calculus
Mathematics Gradient descent and algorithm training both employ multivariate calculus. Derivatives, curvature, divergence, and quadratic approximations will all be covered in class.
Statistics
This is crucial for using classifications in machine learning, such as logistic regression, discrimination analysis, hypothesis testing, and distributions.
Probability
This is crucial for evaluating hypotheses and distributions like the Gaussian distribution and probability density function.
Let's now examine the applications after looking at the various forms of math and data science.
Mostly Used Mathematics in Data Science
Data scientists are essential to businesses' success and daily operations in all sectors. Learning how to use arithmetic in real-world situations will help you comprehend the necessity of data scientists in business and how math is used in those situations.
Let's examine a few examples of how arithmetic is used in real-world settings in current data science and machine learning technologies and applications used by top businesses:
Natural Language Processing (NLP)
NLP uses linear algebra for word embeddings and unsupervised learning methods like topic modeling and predictive analytics. NLP is used in various applications, such as sentiment analysis, speech recognition, language translation, and chatbots.
Computer Vision
For computer vision applications like image processing and representation, linear algebra is also used. When people consider computer vision, self-driving car manufacturers like Tesla come to mind. In order to increase yields in fields like agriculture or better classify diseases and make a diagnosis, computer vision is also commonly employed in these fields.
Marketing and Sales
For purposes like hypothesis testing, marketing and sales statistics help evaluate the success of marketing campaigns. It is also utilized in approaches like causal impact analysis, survey design, and personalized recommendation systems using clustering or predictive modeling to understand consumer behavior, such as why customers are buying from a particular brand.
Get Started with Math for Data Science
Math is a crucial component of a data scientist's education, regardless of the industry you choose to work in after graduation. It guarantees that you can efficiently apply complicated data to address business problems, assist an organization in innovating and solve problems more swiftly.
Use a top online bootcamp provider like Learnbay to ensure you're developing the appropriate skill sets and mathematical capabilities. It provides a complete data science courses in Chennai, which will walk you through all the information you need to learn to pursue a career in data science, including project sessions.