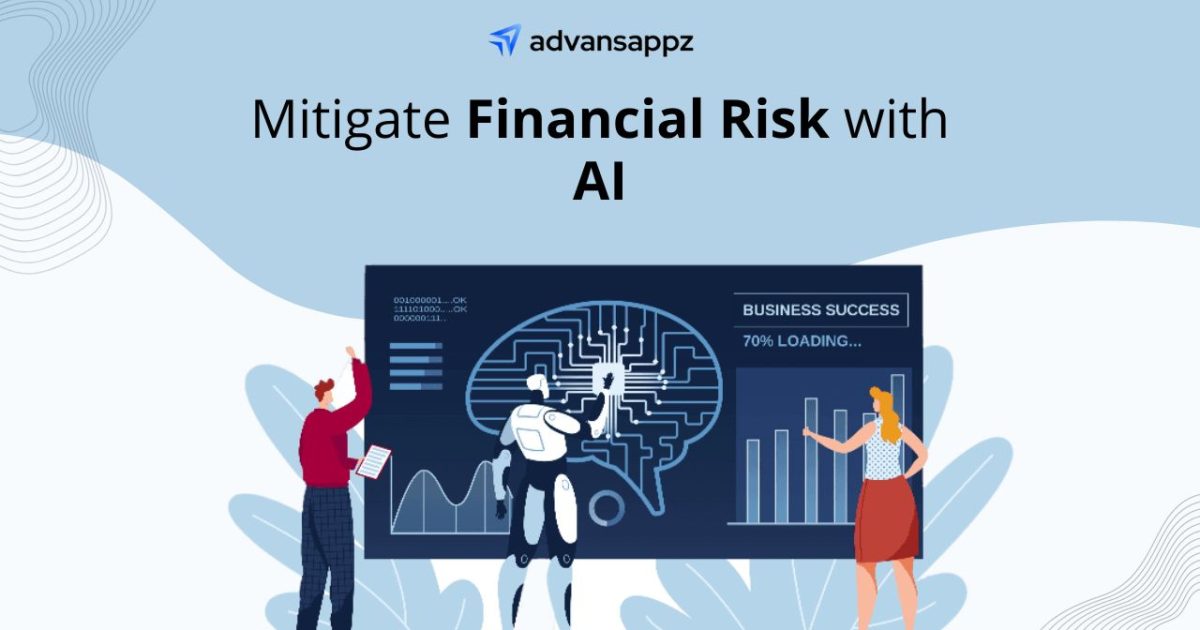
Introduction:
In an increasingly complex and dynamic financial landscape, managing risks has become paramount for businesses and institutions alike. The advent of artificial intelligence (AI) has revolutionized the field of financial risk management, empowering decision-makers with advanced tools and techniques to navigate uncertainty and make informed choices. By leveraging machine learning algorithms, natural language processing, and big data analytics, AI is transforming the way financial risks are identified, assessed, and mitigated. This article explores the multifaceted role of AI in financial risk management, highlighting its benefits and addressing potential challenges.
Enhanced Risk Identification and Assessment:
AI-powered systems excel in the identification and assessment of various types of risks that can impact financial institutions. By analyzing vast amounts of structured and unstructured data, AI algorithms can detect patterns, correlations, and anomalies that may signify potential risks. Traditional risk management approaches often rely on historical data and predefined rules, limiting their effectiveness in adapting to evolving risk landscapes. AI, on the other hand, can learn from new data in real-time and adjust risk models accordingly, providing a more accurate and comprehensive view of potential threats.
Real-Time Monitoring and Early Warning Systems:
Financial markets are dynamic, and risks can emerge rapidly. AI technologies enable real-time monitoring of market conditions, news, and social media sentiments, allowing financial institutions to stay updated on potential risk triggers. By incorporating sentiment analysis and natural language processing techniques, AI systems can process vast amounts of textual data, such as news articles, social media posts, and analyst reports, to assess market sentiment and identify emerging risks. This capability enables early warning systems that alert decision-makers to potential risks promptly, enabling proactive risk mitigation strategies.
Improved Fraud Detection and Prevention:
Financial fraud poses a significant risk to businesses and individuals alike. Traditional rule-based systems for fraud detection have limitations in detecting sophisticated and evolving fraud techniques. AI, with its ability to process vast amounts of data and detect patterns, has revolutionized fraud detection and prevention. Machine learning algorithms can analyze historical data to identify fraudulent patterns and develop models that adapt to new fraud schemes. AI can also integrate multiple data sources, including transactional data, user behavior, and network analysis, to identify anomalies and detect potential fraudulent activities in real-time. This proactive approach not only helps in minimizing losses but also enhances customer trust and loyalty.
Portfolio Optimization and Risk Diversification:
Investment management involves inherent risks, and portfolio optimization is crucial for mitigating these risks while maximizing returns. AI algorithms can analyze historical market data, asset performance, and economic indicators to develop optimal investment strategies. By combining machine learning techniques with advanced portfolio optimization models, AI can generate risk-efficient portfolios tailored to individual investor preferences. These AI-powered solutions consider diverse risk factors, such as market volatility, correlation between assets, and investor risk appetite, enabling better risk diversification and more effective asset allocation.
Stress Testing and Scenario Analysis:
Assessing the resilience of financial institutions under adverse conditions is essential for risk management. AI facilitates stress testing and scenario analysis by simulating various economic scenarios and assessing their impact on the financial health of institutions. By combining historical data with machine learning algorithms, AI systems can model complex interdependencies and analyze how different risk factors interact. These simulations provide valuable insights into potential vulnerabilities and allow decision-makers to devise appropriate risk mitigation strategies.
Regulatory Compliance and Risk Reporting:
The financial industry is subject to stringent regulatory requirements, and ensuring compliance is a critical aspect of risk management. AI technologies can automate compliance monitoring and reporting processes, reducing manual effort and the risk of human error. By analyzing vast amounts of data against regulatory rules and requirements, AI systems can identify potential compliance breaches and anomalies. AI can also generate comprehensive risk reports, providing regulators and stakeholders with accurate and timely information. AI-powered risk reporting systems can consolidate data from various sources, perform data analysis and visualization, and generate customized reports that meet regulatory standards.
Algorithmic Trading and Risk Mitigation:
AI has greatly impacted the field of algorithmic trading, enabling sophisticated trading strategies and risk mitigation techniques. By analyzing market data, AI algorithms can identify patterns and trends that may indicate potential trading opportunities or risks. Machine learning models can learn from historical data and adapt trading strategies in real-time based on market conditions. AI-powered algorithms can also incorporate risk management techniques, such as stop-loss orders and position sizing, to mitigate potential losses. This combination of advanced analytics and risk mitigation strategies enhances trading efficiency and reduces the impact of market volatility.
Challenges and Considerations:
While the integration of AI in financial risk management offers numerous benefits, it is essential to acknowledge and address potential challenges:
- Data Quality and Bias: AI systems heavily rely on data, and the quality and bias in the data can impact the accuracy of risk assessments. Ensuring the availability of high-quality and diverse data is crucial for training AI models and mitigating bias.
- Interpretability and Explainability: AI algorithms often work as "black boxes," making it difficult to understand the reasoning behind their decisions. It is important to develop interpretability techniques to ensure transparency and accountability in risk management processes.
- Cybersecurity and Data Privacy: The use of AI in financial risk management requires robust cybersecurity measures to protect sensitive data and prevent unauthorized access. Compliance with data privacy regulations is paramount to maintain customer trust and avoid legal repercussions.
- Human Oversight and Governance: AI should complement human expertise rather than replace it entirely. Maintaining human oversight and governance frameworks is essential to ensure ethical and responsible use of AI in financial risk management.
Conclusion:
The role of AI in financial risk management is rapidly evolving, transforming the way risks are identified, assessed, and mitigated. By leveraging advanced algorithms and big data analytics, AI empowers decision-makers with real-time insights, early warning systems, and optimized risk strategies. From enhanced risk identification and fraud detection to portfolio optimization and regulatory compliance, AI revolutionizes risk management practices across the financial industry. However, careful consideration of challenges, such as data quality, interpretability, cybersecurity, and human oversight, is necessary to harness the full potential of AI in financial risk management. As AI continues to advance, its integration with risk management processes will become increasingly vital in navigating complex and uncertain financial landscapes.