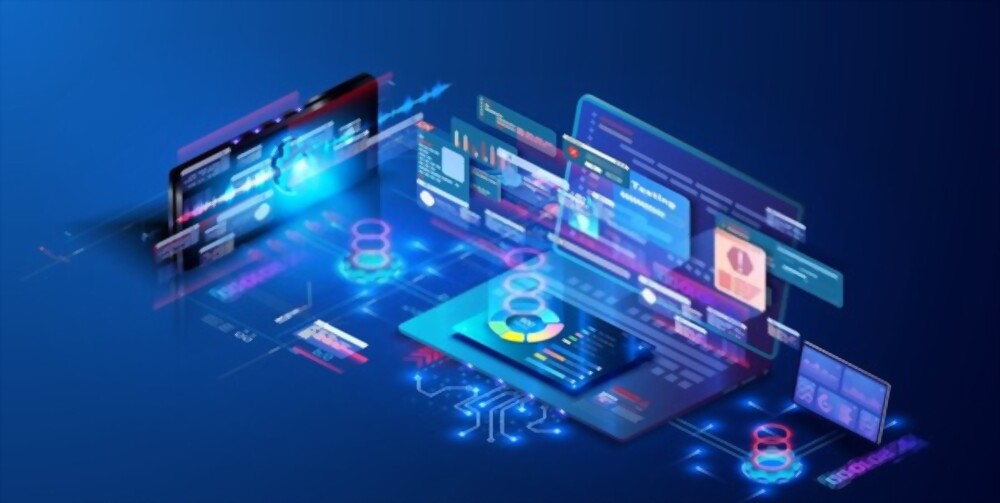
Getting access to real data for model building and software testing can be difficult and expensive. Customer-facing businesses can use privacy-preserving synthetic data to avoid violating their customers' data protection regulations while speeding up their analytics projects.
This type of data can be unstructured, like images and audio, or structured, such as rows and columns of tabular data. It can be created with generative models or by using statistical distributions and simulations.
Artificial intelligence (AI) and machine learning
Synthetic data is generated algorithmically and used as a stand-in for test or production data to validate mathematical models or train machine learning (ML) algorithms. This is useful because real-world data can be difficult to access, expensive, or time-consuming to collect.
It allows organizations to accelerate the pace of development or testing of an AI system and fills gaps where there is insufficient or no existing data. Amazon, for example, uses synthetic data to complete the training of its natural language processing system.
This is especially important for identifying and training on-edge cases, or unique situations that may be impossible to capture using real-world data. Examples include unusual fraud cases or dangerous road accidents that self-driving cars need to be trained on. In warehouses, a similar use case is recognizing and training industrial robots to work with different packages of varying shapes and sizes. In all of these instances, artificially generating the data is faster and more cost-efficient than collecting and processing real-world data.
Banking and Finance
Many financial institutions gather a vast amount of data on their customers. Unfortunately, strict privacy laws and data governance protocols limit how much of this they can use – which limits innovation. Fortunately, synthetic data lets them access high-quality data without putting anyone’s privacy at risk.
Banks can use synthetic data to reduce their risk of fraud, increase lending performance, improve customer service, and develop a more accurate risk model for SMEs. They can also use it to microsegment customers according to value at risk and boost machine learning models.
The best synthetic data generators can synthesize complex time series and transaction data. They can also correct embedded biases and generate realistic test data that is GDPR compliant, statistically representative, and ready to deploy. This allows businesses to save 3 months in evaluating data privacy risks, cut the time to deployment by 4 weeks, and achieve 97% accuracy when training a machine learning model with synthetic data.
Computer vision
Computer vision, the ability to recognize and interpret images, enables many smart systems like self-driving cars or medical imaging. However, vision algorithms need large, correctly labeled datasets to train and improve accuracy. Obtaining this data is time-consuming and expensive, but the synthetic image and video data can speed up model development, testing, and training.
For example, Alphabet subsidiary Waymo generates realistic driving datasets using computer vision to test and train its autonomous vehicle systems. The company’s approach is an efficient alternative to gathering and preparing real-world observations, and it accelerates the time to market for new driver-assist features.
Similarly, Caper, a startup that provides intelligent shopping carts, uses synthetic data to enable its deep-learning models to identify items quickly and accurately in various settings. This allows the company to bring new shopping experiences to stores and deliver better customer service. In addition, it reduces financial costs and algorithm development times for implementing SKU detection in the checkout process
Healthcare
Healthcare is one of the most heavily regulated industries in the world. This is due to complex regulations like HIPAA and GDPR, making it difficult for teams to stay data-driven in the industry.
There are many reasons why real data is unavailable or hard to access, ranging from legacy infrastructures and siloed systems to privacy concerns. Data bias is also a major problem in the healthcare sector, causing misalignment between research findings and reality.
Synthetic Data Generation is a powerful solution to these problems. It’s a method of creating fake data that resembles real-world information while preserving privacy. It can be used for a variety of purposes, including improving machine learning models, completing datasets where there is little or no information, and increasing data accessibility. Moreover, synthetic data can be produced for a wide range of healthcare use cases and does not require secondary consent to be analyzed. This makes it a highly versatile tool for the entire healthcare ecosystem.