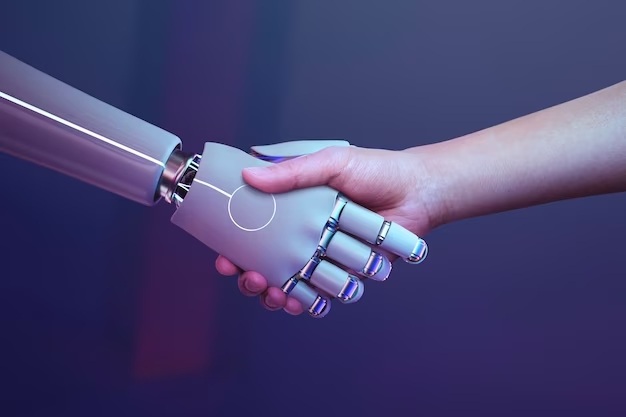
The Evolution of Machine Learning
Machine learning has rapidly evolved over the years, from its early beginnings to its current state where it has become a critical component in various industries. This article explores the historical development of machine learning, the advancements that have shaped its trajectory, and the future prospects of this transformative field.
Machine learning, a subset of artificial intelligence (AI), focuses on developing algorithms and statistical models enabling computers to learn from and make predictions or decisions based on data. This technology has its roots in the mid-20th century, with the groundbreaking work of pioneers such as Arthur Samuel and Alan Turing.
Arthur Samuel's studies on computer checkers in the 1950s laid the foundation for machine learning. He developed a program that improved its performance by learning from previous games, marking the birth of what would later be known as "supervised learning." This breakthrough demonstrated the ability of machines to learn and improve without explicit programming.
However, it wasn't until the 1990s, fueled by advancements in computing power and the availability of large datasets, that machine learning truly started to make significant strides. Researchers began exploring different algorithms and techniques, such as Decision Trees, Support Vector Machines (SVM), and Naive Bayes, which underpin many of the machine learning algorithms used today.
Machine Learning Advancements
In recent years, several key advancements have propelled machine learning to new heights. One of the most significant breakthroughs occurred with the emergence of deep learning and neural networks. Inspired by the structure of the human brain, deep learning algorithms, and neural networks are capable of driving unprecedented levels of accuracy in tasks like image recognition, speech synthesis, and natural language processing. and there are many online machine learning tutorials and machine learning assignment help available for the students.
Additionally, the availability of vast amounts of data, combined with improvements in data storage and processing capabilities, has paved the way for the success of machine learning. Big data allows algorithms to learn from diverse and extensive datasets, enabling more accurate predictions and insights.
Another essential aspect of machine learning advancement lies in the development of powerful frameworks and libraries that streamline the implementation of complex algorithms. Python libraries like TensorFlow, PyTorch, and Scikit-Learn have made it easier for researchers and developers to experiment with and deploy sophisticated machine learning models.
The integration of machine learning into various industries, ranging from healthcare and finance to marketing and transportation, has driven further innovation. Machine learning algorithms are being used to develop personalized healthcare solutions, improve fraud detection, enhance customer targeting, and optimize logistics and transportation systems.
Future of Machine Learning
The future of machine learning is exceedingly promising, as the field continues to push boundaries and tackle new challenges. Here are some key areas of focus that will shape the future of this technology:
1. Reinforcement Learning: Reinforcement learning, which involves training agents through reward-based systems, holds great potential. This approach will likely play a significant role in developing autonomous systems, self-driving cars, and intelligent robots capable of adapting and learning in dynamic environments.
2. Explainable AI: As machine learning models become increasingly complex, there is a growing need for transparency and interpretability. Explainable AI aims to provide insights into how models make decisions, enabling humans to understand, trust, and modify AI-driven systems effectively.
3. Federated Learning: Federated learning enables training machine learning models across multiple devices or edge devices without requiring data to be sent to a central server. This approach addresses privacy concerns while still benefiting from the collective knowledge of diverse datasets.
4. Quantum Machine Learning: With the advent of quantum computing, researchers are exploring how quantum principles can enhance machine learning algorithms. Quantum machine learning has the potential to solve complex optimization problems and significantly speed up computations.
5. Ethical Considerations: As machine learning becomes more ubiquitous, addressing ethical concerns surrounding bias, fairness, and accountability becomes imperative. Ensuring that algorithms are unbiased and do not perpetuate discrimination is a critical aspect of machine learning development.
Machine learning has come a long way since its inception, and its future holds immense possibilities. As researchers continue to explore new avenues, refine algorithms, and address ethical concerns, machine learning will continue to shape our world, revolutionizing industries, and empowering society with transformative capabilities.