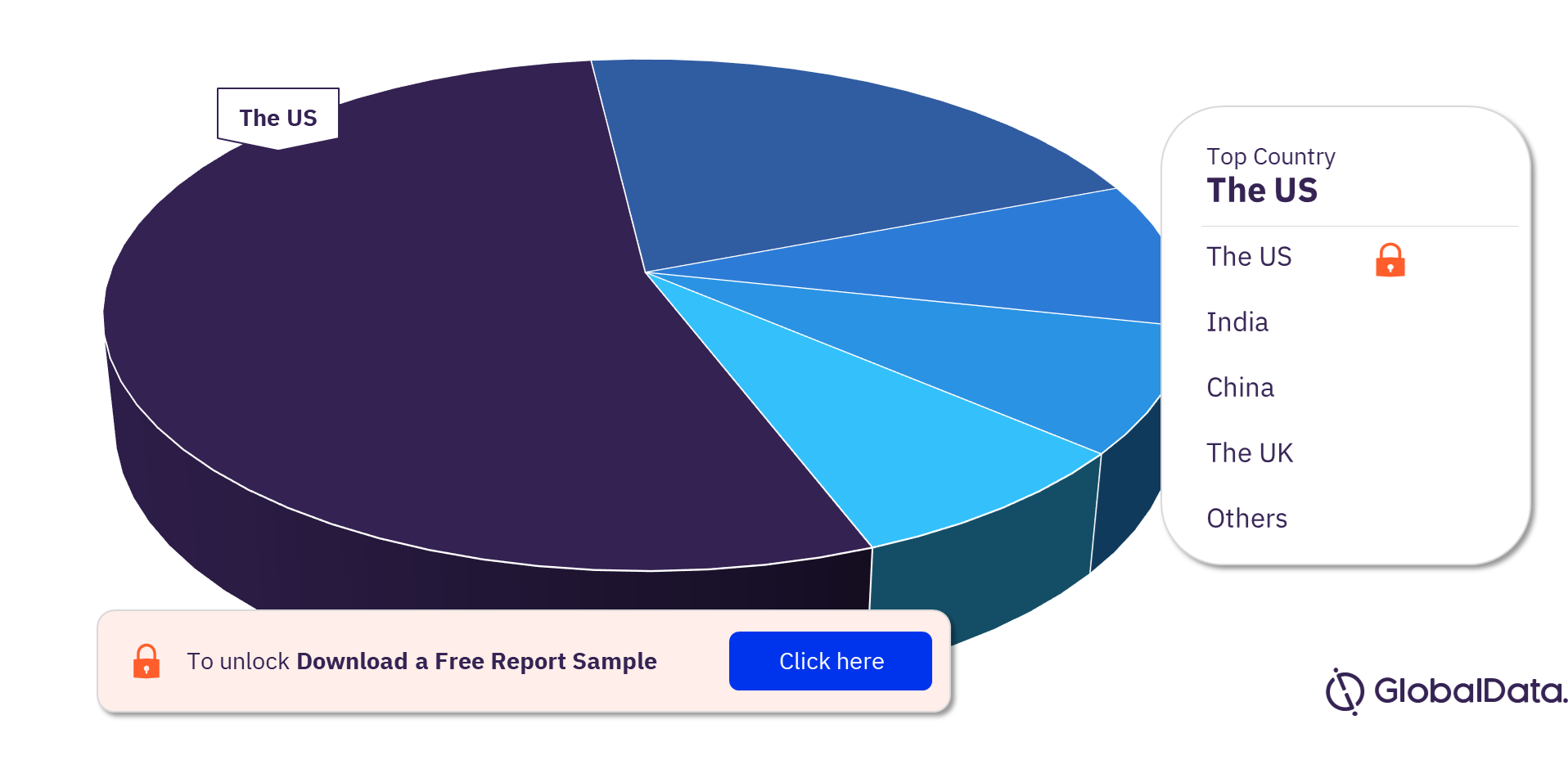
Predicting startup success with an AI model involves analyzing a range of key metrics and data points that provide insights into various aspects of a startup's performance, team dynamics, market positioning, and growth potential.
For more geography insights into the spotting future unicorn predictions, download a free report sample
Here are key metrics and data points that are commonly considered in the development of AI models for predicting startup success:
1. Financial Metrics:
- Revenue Growth: The rate at which a startup's revenue is increasing over time.
- Burn Rate: The rate at which a startup is using its cash reserves.
- Profitability: The ability of the startup to generate profits.
2. Market Analysis:
- Market Size: The total addressable market for the startup's product or service.
- Competitive Landscape: The number and strength of competitors in the market.
- Market Trends: Identifying trends that could impact the startup's growth.
3. Team Competency:
- Founder Experience: The background, skills, and experience of the founding team.
- Team Cohesion: The effectiveness of communication and collaboration within the founding team.
4. Product/Market Fit:
- Customer Satisfaction: Feedback from customers regarding the product or service.
- Retention Rate: The percentage of customers who continue to use the product over time.
5. Customer Acquisition and Retention:
- Customer Acquisition Cost (CAC): The cost of acquiring a new customer.
- Lifetime Value (LTV): The total revenue a startup expects to earn from a customer over their lifetime.
- Churn Rate: The rate at which customers stop using the product or service.
6. Innovation and Differentiation:
- Unique Value Proposition (UVP): What sets the startup apart from competitors.
- Patents or Intellectual Property: Protection of innovative ideas through patents or IP.
7. Social and Online Presence:
- Social Media Engagement: Metrics such as likes, shares, and comments on social media platforms.
- Online Reviews: Feedback and ratings on review platforms.
8. Industry-Specific Metrics:
- Regulatory Compliance: Adherence to industry regulations and legal requirements.
- Technology Trends: Utilization of cutting-edge technologies relevant to the industry.
9. Operational Efficiency:
- Operational Costs: Efficiency in managing day-to-day operational expenses.
- Scalability: The ability of the business model to scale efficiently.
10. User Metrics:
- User Engagement: Time spent on the platform, frequency of usage.
- User Feedback: Direct feedback from users on the product or service.
11. Funding and Investment:
- Funding Rounds: Details of funding rounds, including amounts raised and investors.
- Valuation: The startup's estimated valuation based on funding rounds.
12. Geographic Expansion:
- Expansion Plans: The startup's strategy for entering new markets.
13. Strategic Partnerships:
- Partnership Agreements: Collaborations with other companies or organizations.
14. Legal and Compliance:
- Legal Issues: Any ongoing or past legal challenges.
- Compliance with Laws: Adherence to local and international laws.
15. Customer Demographics:
- Target Audience: Understanding the demographics and needs of the target customer base.
Considerations for Model Development:
- Data Quality:
- Ensure that the data used for training the model is accurate, up-to-date, and free from biases.
- Feature Engineering:
- Identify and create relevant features that capture the nuances of a startup's performance.
- Model Interpretablility:
- Ensure that the AI model provides interpretable insights, allowing stakeholders to understand the factors influencing predictions.
- Continuous Learning:
- Implement mechanisms for continuous learning and model updates based on new data and evolving market conditions.
- Validation and Testing:
- Rigorous validation and testing are essential to assess the model's accuracy and reliability.
- Ethical Considerations:
- Be mindful of ethical considerations, including bias in the data and potential societal impacts.
Emerging Trends in AI-Driven Startup Prediction:
- Natural Language Processing (NLP):
- Analyzing unstructured data such as news articles, social media sentiment, and customer reviews using NLP techniques.
- Explainable AI:
- The emphasis on making AI models more transparent and explainable to build trust among stakeholders.
- Integration with Blockchain:
- Using blockchain for secure and transparent storage of data, especially in cases where data integrity is crucial.
- Dynamic Risk Assessment:
- Incorporating real-time risk assessments and adapting predictions based on evolving risk factors.
- Collaborative Prediction Models:
- Collaborative models that leverage insights from various stakeholders, including industry experts and crowdsourced opinions.
Predicting startup success with AI is a complex task that requires a multidimensional approach, considering various metrics and data points across different facets of a startup's operations. The continuous evolution of technology and data analytics methodologies will likely contribute to further advancements in this field.