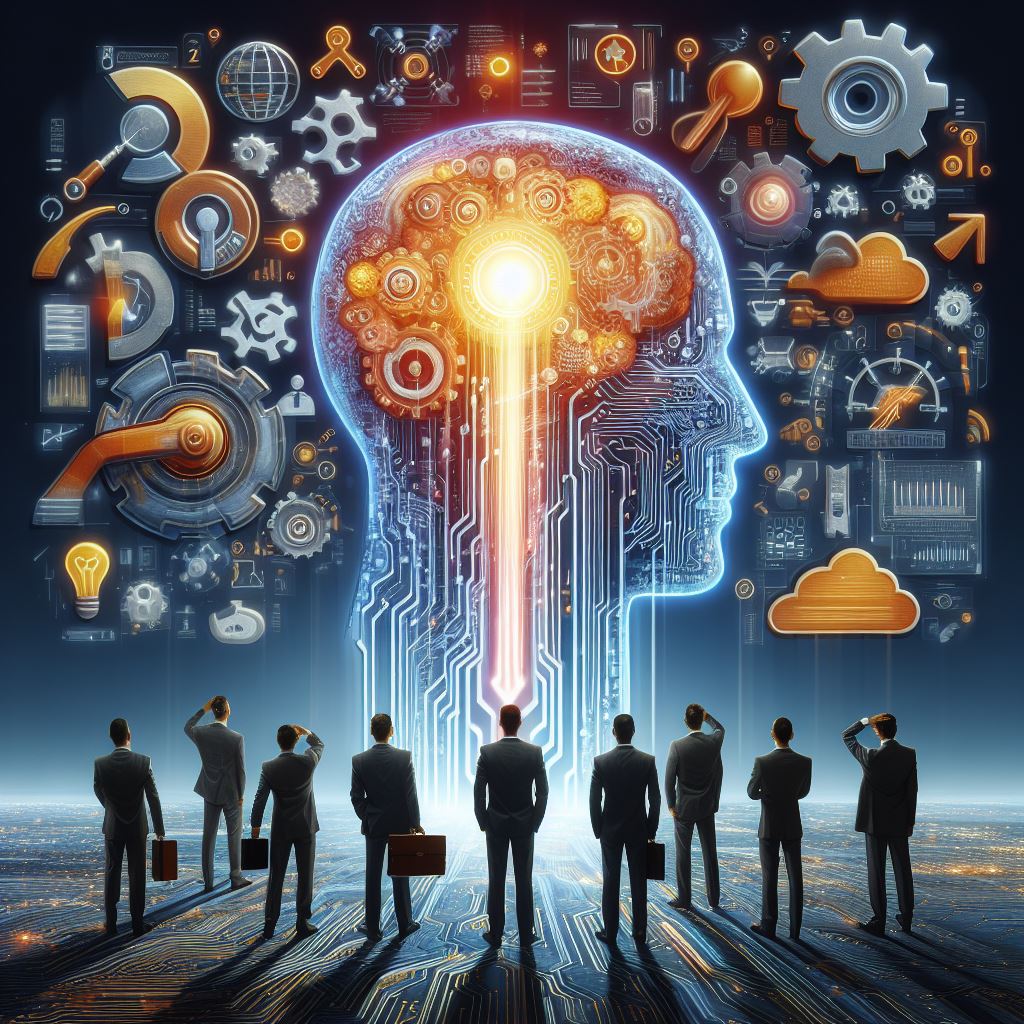
In today's data-driven world, organizations are drowning in information. But within this vast sea lies valuable, actionable insights waiting to be extracted. This is where intelligent data extraction (IDE) comes in. IDE is the process of automatically identifying, classifying, and extracting relevant data from various sources, including documents, emails, forms, and web pages.
Why is Intelligent Data Extraction Important?
Traditionally, data extraction was a manual or semi-manual process, prone to errors and inefficiencies. IDE, powered by Artificial Intelligence (AI) and Machine Learning (ML), automates this process, leading to significant benefits like increased efficiency, improved accuracy, enhanced data quality, faster time to insights and reduced costs.
The Intelligent Data Extraction Process
Here's a simplified breakdown of the steps involved in IDE:
1. Data Source Identification: In the world of intelligent data extraction (IDE), the first crucial step is data source identification. This involves pinpointing the location of the valuable information you seek. This can include various digital formats like emails, invoices, contracts, and webpages. Even physical documents become viable sources through the use of Optical Character Recognition (OCR) technology. By accurately identifying the data source, IDE systems can tailor the extraction process for optimal efficiency and accuracy
2. Data Pre-processing: Data pre-processing is a crucial first step in intelligent data extraction (IDE). This stage involves cleaning, structuring, and preparing the raw data for accurate extraction. Imagine an archaeologist sifting through dirt and debris before uncovering valuable artifacts. Similarly, data pre-processing removes inconsistencies, corrects errors, and organizes the data into a format suitable for AI and ML algorithms to identify patterns and extract relevant information. This ensures the accuracy and efficiency of the entire IDE process, laying the groundwork for reliable insights and informed decision-making.
3. Pattern Recognition: Pattern recognition is the cornerstone of intelligent data extraction (IDE). It acts like a digital detective, sifting through documents, emails, and other sources to identify recurring structures and entities relevant to the extraction task. AI algorithms, particularly Natural Language Processing (NLP), play a crucial role in pattern recognition. NLP allows IDE to understand the context and meaning within data, recognizing patterns like dates, names, addresses, or specific keywords. This empowers IDE to differentiate between relevant information and irrelevant noise, ensuring accurate and efficient extraction of the desired data.
4. Data Extraction: Within intelligent data extraction (IDE), data extraction is the core function, but with a significant upgrade. Traditionally, data extraction involved manually sifting through documents or coding scripts to pull out specific information. IDE automates this process. It leverages AI and machine learning to identify patterns and entities within various data sources – emails, scanned documents, webpages, and more. This allows IDE to intelligently extract the relevant data points you need with far greater accuracy and efficiency compared to manual methods.
5. Data Validation and Cleaning: Within the intelligent data extraction process, data validation and cleaning play a crucial role in ensuring the quality and usability of the extracted information. This stage involves verifying the accuracy and consistency of the extracted data against predefined rules or reference sets. Techniques like anomaly detection and cross-referencing can identify inconsistencies and missing values. Cleaning methods may involve correcting formatting errors, standardizing units of measurement, and filling in missing data through imputation techniques. This meticulous process ensures the extracted data is reliable and ready for further analysis, ultimately driving better decision-making and maximizing the value derived from intelligent data extraction.
6. Data Integration: In the intelligent data extraction (IDE) process, data integration and cleaning play a crucial role in ensuring the usability and accuracy of extracted information. Data integration involves combining data from various sources, like emails, documents, and webpages, into a unified format. This allows for a holistic view of the extracted information. Data cleaning, on the other hand, focuses on removing errors, inconsistencies, and irrelevant data points. Techniques like standardization, deduplication, and anomaly detection ensure the extracted data is high-quality and ready for further analysis.
The Technological Powerhouse Behind IDE
Several technologies work in concert to make intelligent data extraction possible:
- Artificial Intelligence (AI): Artificial intelligence plays a starring role in intelligent data extraction (IDE). AI's subfield, Natural Language Processing (NLP), allows IDE systems to understand the context and meaning within documents. This empowers them to recognize and differentiate between relevant data points and irrelevant information. AI algorithms can also learn and adapt over time, thanks to Machine Learning (ML).
- Machine Learning (ML): Machine Learning plays a crucial role in intelligent data extraction by constantly learning and improving the process. Here's how: ML algorithms analyze vast amounts of data to identify patterns and structures within documents. This allows them to recognize relevant information and differentiate it from irrelevant content. Over time, ML models learn from successes and failures in data extraction.
- Optical Character Recognition (OCR): Optical Character Recognition (OCR) plays a crucial role in intelligent data extraction by acting as the bridge between physical documents and the digital world. OCR technology transforms scanned documents, images, and even handwritten text into machine-readable text. This allows intelligent data extraction tools to analyze the content and identify relevant information.
- Robotic Process Automation (RPA): Robotic Process Automation (RPA) plays a crucial role in intelligent data extraction by automating the repetitive and rule-based tasks involved in the process. This includes tasks like identifying and locating specific data points, copying and pasting extracted data into designated fields, and validating and formatting the extracted data to ensure accuracy and consistency.
Future-proofing with Intelligent Data Extraction
In the age of information overload, intelligent data extraction serves as a key tool to unlock the true value hidden within data. By embracing IDE, businesses can become more efficient, data-driven, and future-ready, ultimately achieving success in a dynamic and competitive marketplace. As technology continues to evolve, IDE will become even more sophisticated, offering even greater opportunities to extract valuable insights and fuel organizational growth.