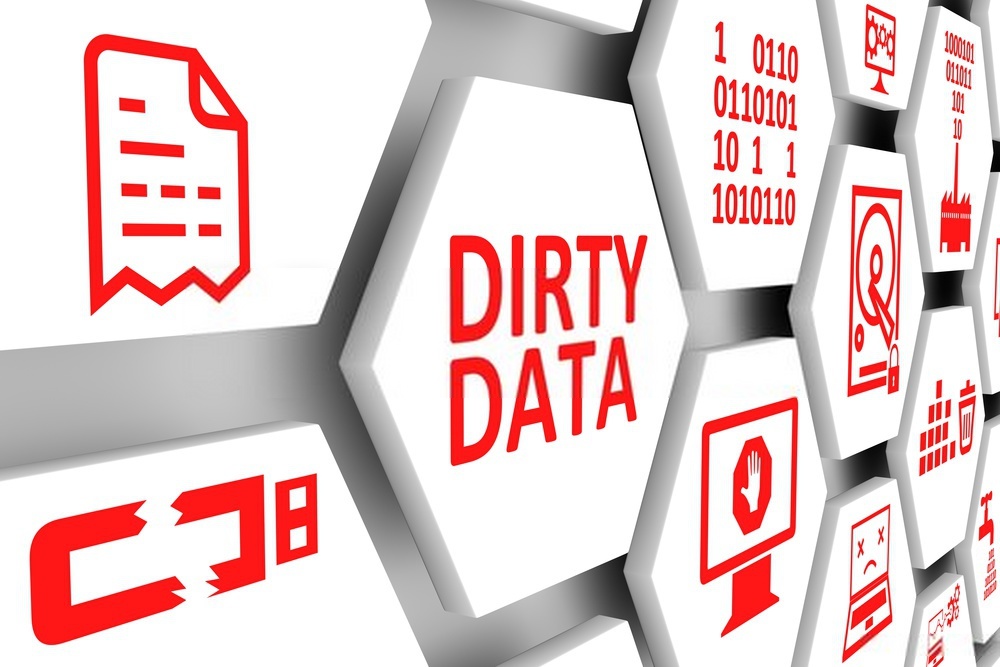
Dirty data is the biggest problem B2B data aggregators are facing today. No wonder poor data costs U.S. businesses $611 billion per year. The fact that only 16% of companies are confident in the accuracy of the data they use, aggravates the need to take data hygiene management on a war footing. The fact that 40% of B2B data sold by aggregators is either invalid, incomplete, or duplicate increases the gravity of the situation.
Only clean and updated data must be sold to clients for them to have effective marketing campaigns and conversions leading to profitable growth. For B2B data aggregators the process starts with identifying dirty data and taking measures to keep it clean and updated in real-time. The best way to address the challenge of dirty data is to find and clean bad B2B data.
Which are 5 common dirty data types?
Tackling dirty data is not as simple as you may think. It involves identifying dirty data and leveraging manual and automated workflows to combat it. Here we will talk about 5 common dirty data types, how to find them and how to clean your database to keep it healthy.
1. Duplicate Data
Duplicate records in your B2B database can reach as high as 20% to 30% hitting your client’s marketing campaigns, customer experience, and defaming you as a B2B data aggregator. Recent studies have shown that 15% of leads that you sell to your clients can contain duplicate data.
- Duplicates creep in your database in multiple ways mostly while transferring records from one data source to another.
- Adding to the complexity of the problem are different kinds of duplicates like exact, partial, variable in the same or multiple sources.
- Customers in your database change their email, job title, etc. and with these differences, a new record is created resulting in a duplicate.
- Name of your customer is saved in different ways and if they are not matched, they again result in the creation of new records.
Identifying duplicate records or deleting them does not suffice. Every record has a unique piece of information like one may have email and the other may have the phone number and both pieces of information are required. The solution lies in matching records with help of smart tooling and techniques. Use of merge/purge workflow to merge records eliminates duplicates and at the same time retains valuable and relevant information.
2. Incomplete Data
One-in-five of your clients lose revenue and customers as a result of the incomplete B2B data you sold them, according to research by Dun & Bradstreet. A survey suggests that 20 % of clients lost a customer sometime - due to incomplete data. B2B data goes beyond contact information.
- The gaps in your B2B database like missing email, phone number, industry, title, last name, etc. can impact your client’s connect ratio with potential leads.
- Your clients today look for data such as demographic, firmographic, chronographic, technographic, behavioral, social media, intent, market intelligence, etc. for accurate targeting.
Investment in automated data enrichment solutions helps efficiently resolve the challenge of incomplete data. It also helps you to enrich your B2B data with additional records. Data harvesting from both public and private sources using programmable bots, web scrapers and crawlers working on pre-determined algorithms works effectively to capture relevant information to enrich your database with accuracy. Taking the assistance of data enrichment experts proves to be a smart move for improving data quality.
3. Outdated Data
Business databases decay faster than a speeding bullet. Marketing Sherpas research shows that B2B data decays at a rate of 2.1% per month. This is an annualized rate of 22.5%. Emails, phone numbers, contact person, etc. change very frequently making your database outdated. It gets difficult to track outdated data as people change jobs, companies merge, people change departments, and so on.
Constant real-time data update is important to keep your B2B data relevant and current. Automated web research techniques are leveraged to capture and enrich records in real-time. Scheduled macros and bots with different time windows continuously gather data to keep modifying and updating your B2B database. Any change in the existing data is tracked by rule-based macros that trigger alerts for appropriate action. Redundant, obsolete, and trivial (ROT) data is flushed out from the database and replaced with current records constantly.
4. Incorrect Data
SiriusDecisions research shows that between 10% and 25% of B2B marketing database contacts contain critical errors. Inaccurate data is the biggest challenge for B2B aggregators making it all the more difficult for the marketing team of their clients. Data collected from the most authentic sources also need validation checks as data decays very fast. Your B2B database needs to be validated and verified using smart tools.
Data validation is a key step in keeping the data accurate and weeing out inaccuracies. Multi-layered technology-enabled quality checks ensure accurate data validation. Rule-based macros and bots make the validation process effective. A series of cross-checks using open source data to authenticate existing database; internal and external sources. Web research and other data sources are used to identify irrelevant, invalid, or obsolete data. Manual, as well as rule-based validation, is used for real-time data validation.
5. Inconsistent Data
Data captured from multiple sources in various formats need standardization to be able to draw actionable insights from it. You collect data from authentic sources, cleanse the data, remove duplicates and outdated data, and use mechanisms to keep it updated in real-time. But if your B2B data is not consistent - it will not fetch the worth when sold to your clients.
Managing database inconsistencies manually is a challenge where technology-enabled solutions are a savior. A well-planned data integration system combines data from different sources providing your clients a unified view of the data. Data standardization services process voluminous, unstructured, and multi-format data in varying formats and help maintain the consistency and reliability of your datasets. ML models can be fed typical data deviations which can help build a database normalization system.
Conclusion
Inefficient data cleansing management allows good and high-performing data into dirty data. It eminently requires professional data cleansing services. It impacts your B2B database business and brand credibility in more ways than one; low-performing marketing campaigns of your clients, poor customer experience for you resulting in lost revenue, and much more.
Constant data audits identify the presence of dirty data in your database which needs correction through cleansing. Setting up an infrastructure, upgrading technology, and hiring skilled resources may not be a viable option for B2B data aggregators to keep their data clean. The best way to keep data clean and maintain data hygiene is to opt for assistance from data cleansing experts.