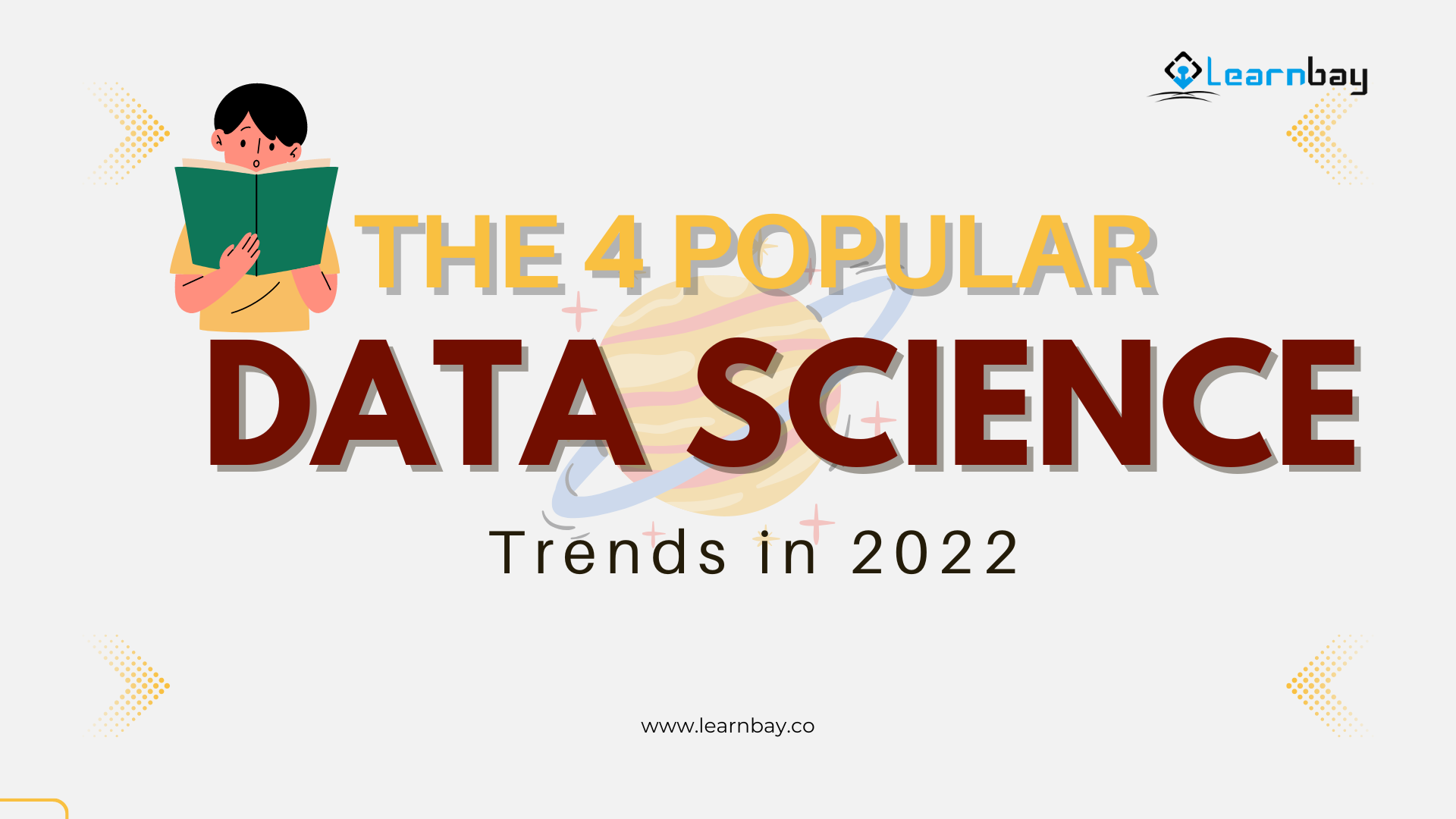
The emergence of data science as a field of study and practical application over the last century has resulted in the development of technologies such as deep learning, NLP, and computer vision. In general, it has facilitated the emergence of machine learning (ML) as a method of working towards artificial intelligence (AI), a field of technology that is rapidly transforming the way we work and live.
Big Data, predictive analytics, and artificial intelligence are examples of data science concepts with both theoretical and practical applications. If data is the information age's oil and machine learning is the engine, then data science is the digital domain's equivalent of the physical laws that cause combustion and pistons to move.
Data Science Trends
A key point to remember is that as the importance of understanding how to work with data grows, so does the science behind it. It was considered a niche crossover subject straddling statistics, mathematics, and computing ten years ago and taught at several universities. Its significance in the world of business and commerce is well established today, and there are numerous paths, including online data science certification courses in Mumbai and on-the-job training, that can equip us to apply these principles. This has resulted in the much-discussed "democratization" of data science, which will undoubtedly impact trends discussed in 2022 and beyond.
TinyML and Small Data
Big Data refers to the rapid increase in the digital data we generate, collect, and analyze. But it's not just the data that's large; the ML algorithms we use to process it can also be quite large. GPT-3, the most complex and largest system capable of modeling human language, has approximately 175 billion parameters.
This is fine if you're working on cloud-based systems with unlimited bandwidth, but it is far from covering all the use cases where ML can add value. As a result, the concept of "small data" has emerged as a paradigm to facilitate rapid, cognitive analysis of the most critical data in situations where time, bandwidth, or energy expenditure are crucial. It is closely related to the idea of edge computing. When attempting to avoid a traffic collision in an emergency, self-driving cars, for example, cannot rely on being able to send and receive data from a centralized cloud server.
TinyML refers to machine learning algorithms designed to take up as little space as possible to run on low-powered hardware near the action. By 2022, it will be found in many embedded systems, including wearables, home appliances, automobiles, industrial equipment, and agricultural machinery, making them more innovative and valuable.
This is about how businesses use our data to provide us with increasingly valuable, worthwhile, or enjoyable experiences. This could imply less friction and hassle in e-commerce, more user-friendly interfaces and front-ends in the software we use, or spending less time on hold and being transferred between departments when we contact customer service.
Deepfakes, generative AI, and synthetic data are all examples of Artificial Intelligence
When terrifyingly realistic "deep fake" videos went viral this year, many of us were duped into thinking Tom Cruise had started posting on TikTok. The technology behind this is known as generative AI, and it aims to generate or create something that does not exist in reality, in this case, Tom Cruise regaling us with tales of meeting Mikhail Gorbachev. The arts and entertainment industries have quickly embraced generative AI, with Martin Scorsese de-aging Robert DeNiro in The Irishman and (spoiler alert) a young Mark Hamill appearing in The Mandalorian.
Convergence
AI, the internet of things (IoT), cloud computing, and ultrafast networks such as 5G are the foundations of digital transformation, and data is the fuel that powers them all. All these technologies exist separately, but they can do much more when combined. Artificial intelligence enables IoT devices to act intelligently, interacting with one another with as little human intervention as possible, resulting in a wave of automation and the creation of smart homes, smart factories, and smart cities. 5G and other ultra-fast networks not only enable faster data transmission;
AutoML
Acronym for "automated machine learning." As stated in the introduction, AutoML is an exciting trend that hastened the "democratization" of data science. It is aimed at experts whose specialized knowledge and insights put them in an ideal position to develop solutions to the most pressing problems in their respective fields. However, they frequently need more coding knowledge to apply AI to those problems. AutoML solution developers hope to create tools and platforms that anyone can use to build their ML apps.
A large portion of a data scientist's time is frequently spent on data cleansing and preparation - tasks that require data skills and are often repetitive and mundane. AutoML involves automating those tasks at its most basic, but it is increasingly also about developing models, algorithms, and neural networks.
Hope you enjoyed reading this article on top data science trends. Join the remarkable data science course in Mumbai to reinvent your career in this exciting field and land dream positions in MAANG companies.