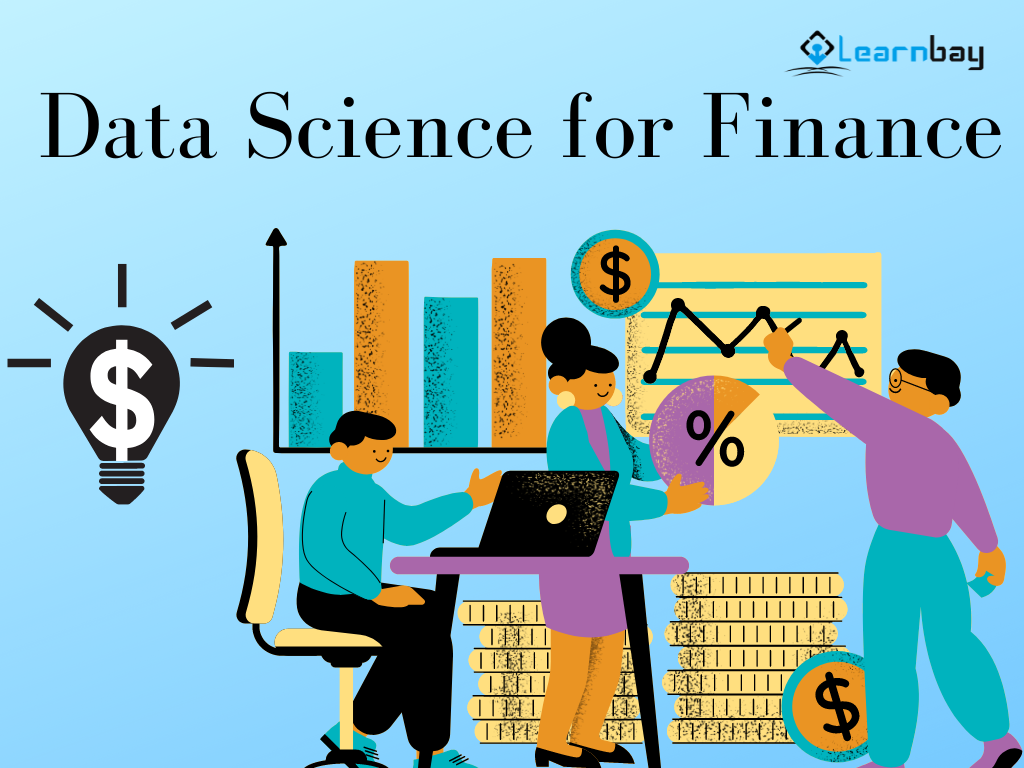
Data science is an area of study that works with massive amounts of data using cutting-edge technology tools and methodologies to identify patterns and extract valuable information that can be used to make business and financial choices. To create structured and useful data, it employs sophisticated machine learning techniques.
What is Data Science?
Data Science is the application of advanced analytics, statistics, and scientific concepts to extract valuable information from structured or unstructured data and draw useful conclusions. Thus, they are useful for making corporate decisions, developing strategy, and other tasks like financial planning.
Data science frequently makes use of concepts like artificial intelligence and machine learning. Artificial intelligence mimics human intelligence, which focuses on comprehending historical data and making meaningful inferences using machine learning. Today, data science is critical in banking and finance. Data science practitioners are in high demand in these areas because many businesses have begun to integrate data science into their organizational and decision-making processes. The Learnbay data science certification course can be a springboard for your career development if you wish to become a financial data scientist.
Finance Data Science:
Data science is equally important and applicable in the financial industry as it is in other commercial decision-making domains. The appropriate teams within the organization, particularly the investment and financial team, benefit from the expertise in data science for financial assistance by working with them to develop tools and dashboards to enhance the investment process.
What Roles Do Data Science and Finance Play?
Financial data scientists are particularly interested in the issues faced by the finance industry. Data science may help the financial sector understand current trends and make decisions based on them. Data science and finance are based on using state-of-the-art statistical machine learning algorithms to organize data sets and produce financial insights.
It involves taking calculated risks to increase earnings. Working in data science necessitates both technical skills and subject knowledge.
Advantages of Data Science in Banking:
The field of data science in finance is rapidly increasing and offers numerous advantages. You can learn how data science contributes to finance and what advantages it has by reading the points below:
Increased Sales and Income:
Several financial institutions recognize the need of expanding client connections with meaningful human ties. Businesses can use data science to determine whether or not their customers are using improved amenities. It contributes to a completely new level of customer experience personalization. Financial data analysts can investigate consumer behavior and generate insightful data that allows various organisations to give the finest services to their clients in real-time. Financial businesses can better predict future client behavior and value production using this knowledge.
Obtaining Useful Information:
Fraud is a significant issue in financial organizations. Fraud and cybercrime have expanded dramatically as the country's transactions have increased. Organizations are vulnerable to fraud as a result of proactive and predictive analysis. With today's data-driven financial systems, abnormalities are there, which reduces the harm. Reliable clients with verified purchase histories are among the data-driven fraud study discoveries that can benefit the company and lower the risk of fraud.
Robotic Process Automation-Based Routine Optimization:
Businesses spend a lot of time on routine tasks and reconciliations, which adds up to hundreds of hours per year for accountants. By comparing transactions across several data sources, data scientists in finance can free up time and resources for tasks that are more important. Robotic process automation reduces risk assessment and creditworthiness by transparently presenting results regarding customer data. Data science can be used by any bank or financial institution to streamline processes and increase performance. Examine the data scientist course fees that are available.
Data Science Applications in Finance:
There are numerous applications of data science in finance, which increases the significance of finance in data science. A few of the numerous data science applications in finance are listed below:
Risk Assessment:
Every business that conducts operations runs a risk of some kind. Today, every company must do a threat and risk assessment. Risk analytics refers to this strategic action. Financial professionals must apply data science when assessing risk. Data science implementation in the financial markets can help a company increase its efficacy and security because data is the cornerstone of risk management. Data science includes the study of approaches for solving problems.
Real-time Analytic:
The main disadvantage of typically evaluating data in batches instead of in real time was that the data would be outdated by the time it was examined. Indeed, it became historical data, and judgments based on past data are no longer valid for use with current business modules. Dynamic data pipelines and technology advancements have simplified the process of evaluating data in real time and providing firms with access to data dependent on the scenario.
Consumer Research:
Several firms place a great priority on client personalization. Data scientists can acquire real-time insights into consumer behavior using data analytics in the financial industry, allowing them to make better strategic business decisions. The insurance sector is a prime example of consumer personalization through data science and finance. They use data science to analyze consumer activity patterns to cut losses and determine a consumer's lifespan.
Handling of Customer Data:
Big data's impact on financial institutions' operations has completely changed data science. Data science for financial modeling has altered due to social media inclusion and a big volume of financial transactions. Structured data and unstructured data are the two types of data that are generally obtained. Structured data is already in a specific format, making it easy to analyze and utilize. Unstructured data is not already in a specific format, making analysis more difficult and time-consuming.
Finding financial fraud:
The chance of fraud increases as transaction volume increases. But financial institutions can better track scams and frauds using big data and its analytical tools. Also, the alerted financial institutions conduct investigations right away if any suspicious trade patterns are discovered with the aid of various machine learning algorithms.
How to Start a Financial Data Science Career? [Step-by-Step]:
These are some steps you could take if you want to work as a financial data analyst:
Step 1- Study toward a bachelor's degree:
The first and most fundamental step towards becoming a financial data analyst is earning a bachelor's degree. A bachelor's degree in a discipline with a focus on mathematics is required. You can study one of the following fields for your bachelor's degree:
- Statistics in Mathematics
- Engineering
- Physics, Economics, and Computer Science
Learn about data science course fees.
Step 2- Acquire programming language skills:
You need to be proficient in various programming languages to be a data scientist. You can evaluate and streamline data more effectively if you work using compiled and integrated computer languages. The following programming languages are available for study:
- Python
- C, C++
- Java
- MATLAB
Step 3- Get database knowledge:
In organizations, data is retrieved and stored via databases. The financial data scientists who are doing this work. A range of Relational Database Management Systems must be mastered by you (RDBMS). You could study how to use the following RDBMSs:
- MySQL
- SQL Server
- PostgreSQL
Step 4- Master handling of series data:
You must develop the ability to manage huge data sets, especially those derived from financial streams. Another crucial element you should understand as a financial data scientist is the organization of raw data. You will be able to advise the groups on financial concerns more effectively in this way.
Step 5- Take master's courses:
You might think about earning a master's degree if you want to become an expert in financial concerns. A master's degree or MBA will open up more options for you and position you for leadership positions. It is advised to have a master's degree in statistics or finance.
Conclusion:
Enroll in the Learnbay data analytics course if you want to learn more about data analytics or if you want to improve your current data science abilities. With this training, you can further your professional career and have greater employment possibilities.